

Random probability sampling: where each unit within the overall dataset has the same chance of being selected. Each large sample can then be broken down by a data set. Sampling is when, instead of analyzing an entire dataset, you select a sample or "section" of the data to evaluate. FullStoryįullStory is a Digital Experience Intelligence platform combines rich analytics, robust session detail, and collaboration tools to help you answer questions, understand issues, and uncover opportunities-and then put that knowledge to work. Oracle supports any kind of data model and has different product editions depending on your needs. The integrated cloud applications and platform by Oracle that provides a relational database management system. AWSĬloud services by Amazon that provide services in the form of building blocks that can be used to create and deploy any type of application in the cloud. Store your data in Google Cloud in any file or format. Examples for analytics tools to start with: Google CloudĬloud computing services by Google. Google Analytics is a popular tool used by data analysts and data scientists to gather quantitative data from various sources.Īlongside Google Analytics, there are other tools that can be integrated with internal databases, data lakes, cloud storage, business apps, social media, and IoT devices. There are many methods for collecting data: analytic tools, sampling, questionnaires, and open-source datasets, among others. Ratio: Your height and weight cannot equal zero or be below zero. Interval: A certain temperature can fall below 0 degrees. The difference between interval vs ratio data sets: The ratio scale has an absolute zero or character of origin. Ratio data has all the characteristics of an interval scale, but it can also calculate ratios-meaning you can leverage numbers on a scale against zero. Ratio variables, on the other hand, never fall below zero. Interval scales can represent values below zero. The difference between interval and ratio data derives from their ability to fall below zero. Additionally, continuous data can be classified as interval data or ratio data. The temperature of the sun is 10,000 FahrenheitĪs you can see, these can change at any given time. It differs from discrete data in that it can change its value.Ĭontinuous data can also fluctuate over time. The how many cars driving above the speed limit on a freewayĬontinuous data is data that can take any value and can be infinitely broken down into smaller parts. For instance, the number of students in your class is discrete data to be counted as a whole (for example, you can’t have 22.5 students). Typically, it involves integers-whole numbers, rather than fractions. This type of data only has a certain number of values. The results obtained are objective in nature, so they are extremely accurate.Īn example of a bar graph representing quantitative dataĭiscrete data is information that is collected and can be counted. And due to its numerical nature, there’s less potential for bias. Since quantitative data is statistically analyzed and mathematical in nature, research can be extremely detailed. Knowing what type of quantitative data - also called numerical data - you’re working with helps teams apply the correct type of statistical analysis. Quantification of qualitative data: how a categorical group of people felt about a quiz. Sensors: a photosensor detecting the presence of light. Measurements, counts, and calculations: for example, the attendees of a webinar. The different categories of quantitative data include: Quantitative variables can tell you "how much," "how many," or "how often." It’s data that can be counted or measured, and given a numerical value.
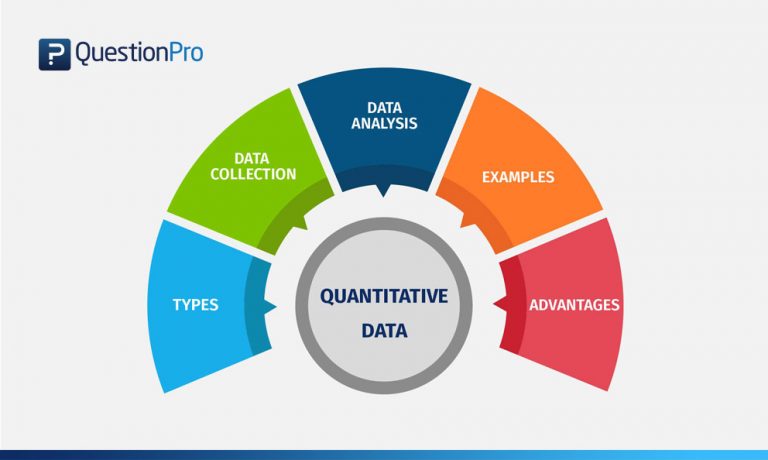
What is quantitative data?Īt its simplest level, quantitative data is information that can be quantified.
#QUANTITATIVE DATA ANALYSIS PROGRAMS HOW TO#
We’ll cover the basics of quantitative data, quantitative data examples, its different types, use cases, and how to collect it for yourself. In order to analyze and interpret this data type, you’ll need to understand the nuances, details, and other factors. Data can be complicated, and the shortcomings and victories aren’t always apparent-they’re buried in the details.ĭata-specifically quantitative data-is no different. Understanding data is a lot like understanding the complexities of life.
